UNRAVELING BUSINESS INTELLIGENCE: NAVIGATING THE COMPLEX PATH TO DATA-DRIVEN SUCCESS
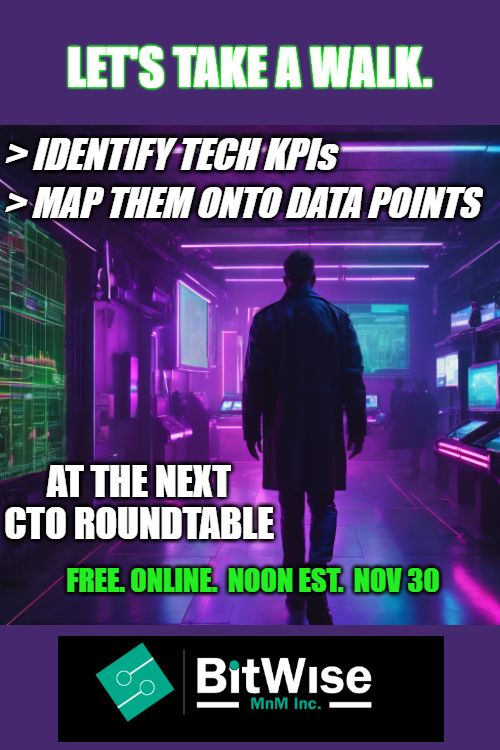
By Mordechai Danielov and Anna Garleff – January 12, 2024
Business intelligence (BI) is not a new concept; it has been an integral part of business strategy for as long as commerce has existed. However, in our rapidly evolving business landscape, the importance of BI has reached new heights, making it a topic of renewed interest and discussion.
In an era dominated by data, businesses are increasingly relying on information to make informed decisions. The need for BI has never been more critical, as organizations strive to harness the power of data to drive success. BI is not just the domain of the IT department; it’s a key player in shaping the destiny of every department within an organization.
CTOs play a pivotal role in KPI development by leveraging their tech expertise to align data metrics with their organization’s strategic goals. Their skill in data modeling is critical to ensuring that KPIs map accurately onto real-world concepts. The CTOs who work collaboratively with stakeholders to identify actionable indicators are the most successful.
This is a part of the process that is frequently missed, skipped over or misunderstood by many CTOs. Only after successful communication with the humans determining the KPIs can the CTO then employ logic to break down abstract ideas into quantifiable representations. By focusing on database audits, you can enhance data source clarity, address redundancies and improve data quality. In this way, CTOs bridge the gap between technical and business teams, and facilitate the creation of flexible, durable, and expressive BI tools that empower data-driven decision-making at every level.
ARTICULATING KPIS: THE CHALLENGE AND SOLUTION
One of the major hurdles in BI lies in articulating Key Performance Indicators (KPIs). Deciding what to track and how to display it can be a complex task. The challenge often lies in differentiating between covariance and causation, determining what to display on the dashboard, and what to reserve for drilldowns.
Addressing this challenge requires a deep understanding of business decision-making processes. It’s not just about showing data; it’s about presenting meaningful insights that facilitate actionable decisions. Clear communication and collaboration between IT and business units are paramount in creating BI tools that truly add value.
Consider a scenario in an e-commerce business where the goal is to optimize the conversion rate. Two potential KPIs are identified: the number of website visitors and the number of completed purchases. Now, there’s a need to differentiate between covariance and causation.
On the dashboard, displaying both the number of visitors and completed purchases might seem straightforward. However, if the primary goal is to discern the factors influencing the conversion rate, it becomes crucial to identify the causal relationship. Does an increase in website visitors directly lead to more purchases, or is there an intermediary factor at play?
To address this, a CTO might reserve the detailed causative analysis for a drilldown. By examining specific user behaviors, such as time spent on product pages or interaction with promotional elements, the CTO can uncover the causal factors affecting the conversion rate. This strategic differentiation on the dashboard versus drilldown ensures clarity and actionable insights without overwhelming the primary display with excessive information.
In other words, the KPIs are refined to the most impactful and actionable metrics, ensuring that the dashboard provides a concise overview while allowing for in-depth analysis when necessary. Decision-makers can then allocate resources and efforts based on a precise understanding of what truly drives the desired outcome—optimizing the conversion rate in this case.
DATA SOURCE CLARITY: THE ROLE OF DATABASE AUDITS
Not all companies have a clear understanding of where their data is coming from. A database audit, such as the process BitWise MnM has developed, can reveal redundant, missing, or improperly used data sources. This not only enhances data quality but also ensures that organizations can take actionable steps to improve the BI process.
Smaller organizations might balk at the investment of time and money into a data audit. Here are some factors to consider:
-
Data Quality Impact: A data audit can significantly enhance data quality, leading to more accurate and reliable insights. If your organization heavily relies on data-driven decision-making, ensuring the integrity of your data sources is paramount. Higher data quality often translates into better ROI on your analytical investments.
-
Risk Mitigation: Identifying and rectifying issues such as missing or improperly used data can mitigate risks associated with inaccurate reporting and faulty analyses. In industries where compliance and regulatory adherence are critical, a data audit becomes an essential risk management practice.
-
Operational Efficiency: Reducing redundancy and ensuring data sources are appropriately utilized can enhance operational efficiency. Streamlining your data sources can result in faster processing times, quicker access to information, and a more efficient data infrastructure.
-
Cost of Errors: If your organization has experienced significant financial or operational repercussions due to errors in data, the cost of these errors may outweigh the investment in a data audit. Preventing such errors through proactive auditing can save costs in the long run.
-
Strategic Decision-Making: If your organization relies on data for strategic decision-making, the insights gained from a data audit can be invaluable. It ensures that the data used to inform strategic initiatives is accurate and reliable, contributing to better-informed decisions.
-
Technology Investments: If your organization has invested in advanced analytics, machine learning, or AI, the effectiveness of these technologies is contingent on the quality of input data. A data audit can optimize the performance of these technologies, maximizing the return on your technology investments.
MAPPING PIs TO DATA POINTS: THE IMPORTANCE OF DATA MODELING
Data modeling, a discipline in decline, plays a crucial role in mapping real-world concepts to data points. At BitWise MnM, we know that a robust data model is essential for creating flexible, durable, and expressive BI tools. Only with precise mapping of business processes to data entities can you actually detect overall patterns and make informed decisions. The process of data modeling aligns the data structures with organizational objectives, and actually provides flexibility for evolving business needs.
Effective data models integrate disparate sources, encouraging collaboration and providing a holistic view of the data landscape. Well-structured models enable efficient data retrieval and analysis, reducing ambiguity in KPI interpretation. Ultimately, data modeling serves as the foundation for bridging business goals with actionable metrics, facilitating a streamlined, data-driven approach to decision-making.
CTOs COMMUNICATING WITH STAKEHOLDERS: LEADING BY EXAMPLE
Effective communication between Chief Technology Officers (CTOs) and business stakeholders is vital for successful BI implementation. Leading by example, practicing active listening, and adopting a cooperative approach can bridge the communication gap. It’s about creating a shared understanding that fosters collaboration and informed decision-making.
Instead of overwhelming non-technical stakeholders technical details, ask to hear their perspectives on the critical metrics that matter most to the organization’s strategic objectives. Are there specific areas or outcomes they’d like to prioritize in the data analysis? Their insights should guide your focus and ensure that the KPIs chosen reflect a shared vision for success.
QUANTIFYING SHAREHOLDER VALUE: LOGIC AND VISUAL CLUES
Quantifying shareholder value is challenging but essential. Logic and data representation play a key role in breaking down abstract concepts into quantifiable indicators. Positive and negative indicators, backed by actual data, offer visual clues that help organizations gauge their performance in providing value to shareholders.
The goal is to convert abstract concepts into quantifiable indicators by establishing a structured framework for understanding and measurement. It is essential to identify the relationships between different elements of an abstract concept and translate qualitative aspects into measurable units. For example, in assessing customer satisfaction, logic helps define the variables impacting satisfaction, and data representation allows for the conversion of sentiments into numerical scores, enabling a quantitative evaluation. The synergy between logic and data representation is fundamental in bringing clarity and measurability to abstract ideas.
But be aware: There’s no one-size-fits-all approach to setting KPIs. Every business has unique needs, and KPIs should align with operational requirements. It’s not about telling businesses what to measure but helping them identify what is important and necessary for their success.
Furthermore, helping decision-makers to articulate their KPIs is a process that requires creativity and iteration. Stakeholder education, communication mapping, and database audits are integral steps in ensuring a holistic approach to KPI definition.
OKRs and KPIs: TRACK RESULTS IN DAYS NOT MONTHS
Objectives and Key Results (OKRs) help establish high-level, measurable goals for organizations by establishing ambitious targets and outcomes that can be tracked over the quarter. OKR has been around since the 1970s, and the concept was created by Andy Grove, but popularized by John Doerr, one of the earliest investors in Google.
With OKR, a goal isn’t just what you want to achieve; it must include a way to measure achievement. Embraced by tech companies, OKR can help organizations stay on track in a fast-paced, ever-changing industry, while still encouraging innovation.
OKRs and KPIs may seem similar on the surface, but they both address different aspects of organizational performance. KPIs are focused on the performance of employees, creating goals to measure their success in their careers and within in the organization, whereas OKRs are focused on the organization, helping companies identify quarterly goals to improve business performance and grow the organization’s success. Something for a CTO to think about.
You don’t need to abandon KPIs for OKRs, but you should leverage the differences between the two. OKRs should be tied to business goals and objectives, rather than employees’ work. KPIs on the other hand can be tied directly to an employee’s day-to-day work and performance. Both tools are designed for achieving success in the workplace, each with a different target.
STARTING THE KPI JOURNEY: A CONTINUOUS IMPROVEMENT APPROACH
Starting the journey towards articulating KPIs doesn’t have to be perfect from the outset. It begins with actionable indicators, continuous improvement, and iterative refinement. CTOs oversee the leverage of data discovery, AI, and data scavenging, so that their organizations can evolve their KPIs over time. That’s how KPIs remain relevant and reflective of organizational goals.
In conclusion, the path to successful business intelligence is paved with clear communication, collaboration, and a commitment to continuous improvement. By understanding the challenges, embracing creativity, and leveraging the right tools and methodologies, businesses can unravel the complexities of BI and pave the way to data-driven success.
And always, always be BitWise.
We like people, so feel free to call or write. Humans only.